Becoming an AI-native Company
Everyone today is talking about AI skills. Companies want to hire people who know how to use AI. They expect it in interviews. They hope it will show up in the work. From recruitment conversations to measuring employee performance, output and org productivity, AI is quite the topic of debate at the moment.
Recently companies like Shopify and Duolingo shared publicly how they plan to become more AI-native. Interesting that both of them were quite careful in landing the message softly with the “We deeply care about humans in the age of AI” bookend.
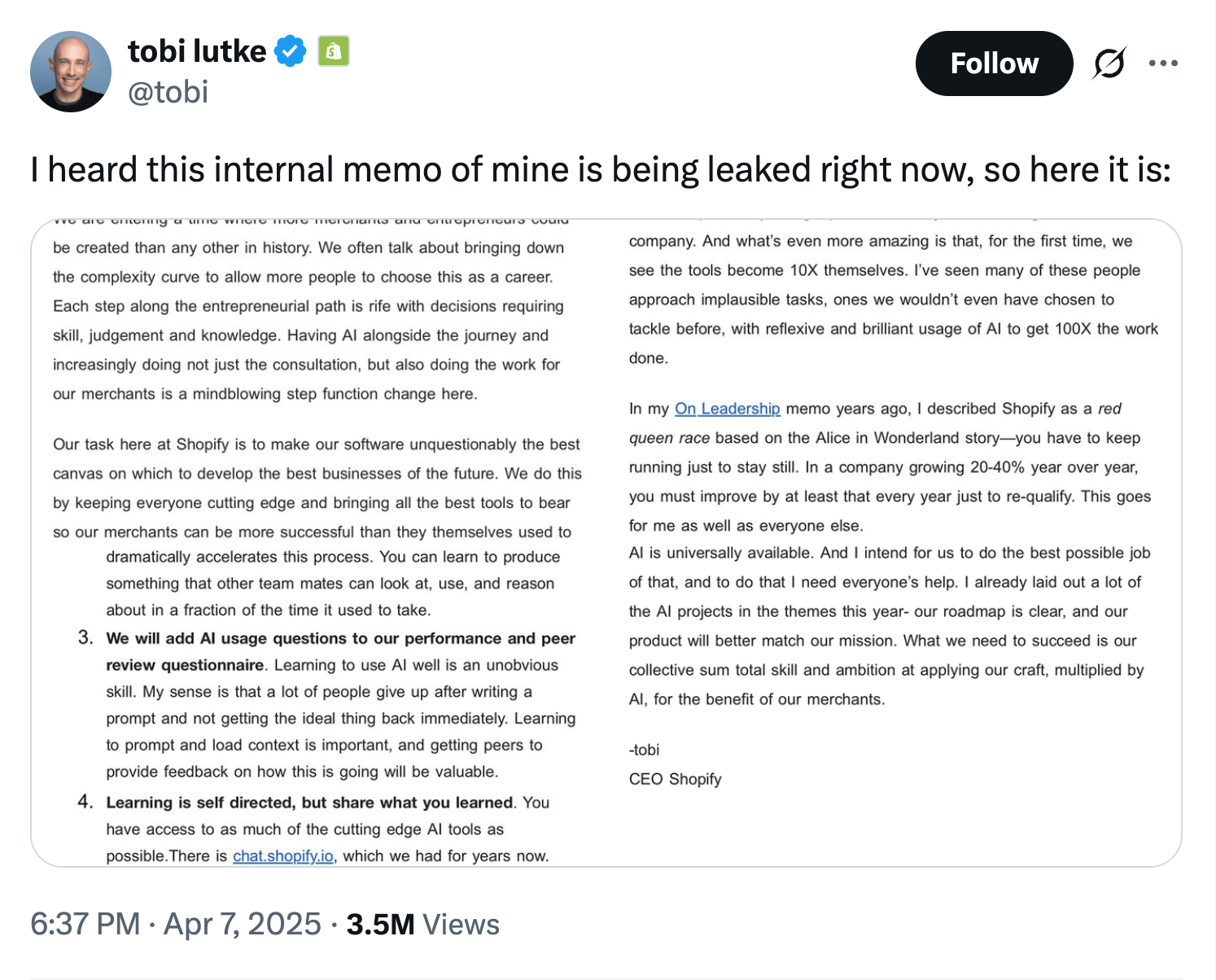
We’ll see this with more companies going forward. Using AI is quickly becoming as synonymous as knowing basic office software. Think Google Docs, Figma, Notion and so on.
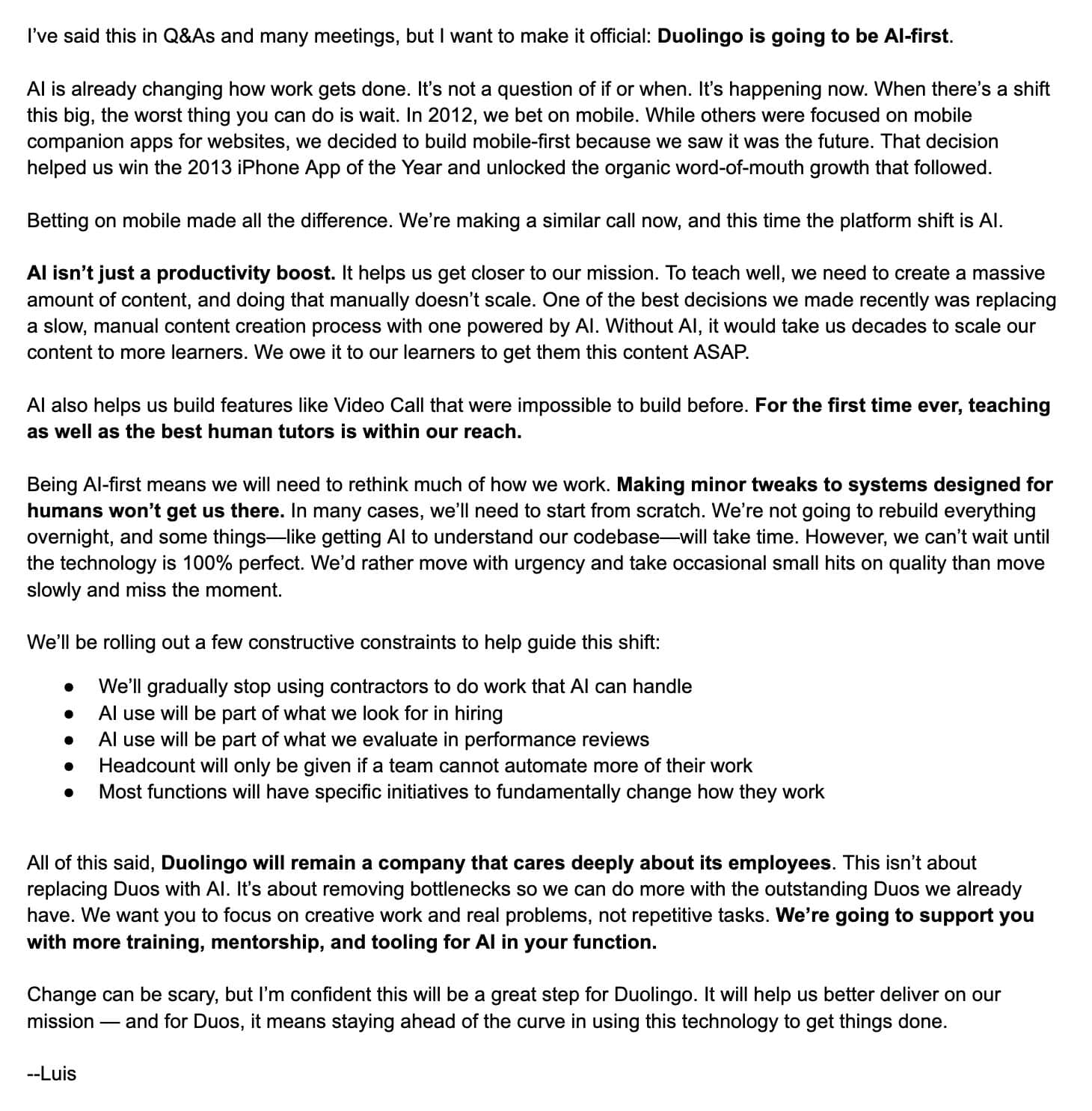
But it’s not enough to expect employees to adopt AI. Companies themselves must become AI-native in how they work, grow, and scale. And above all, companies need to support employees through the change
There won’t be one single playbook that works for all companies. But there are definitely themes that will be common across them and are worth talking about.
Let’s explore a few shifts companies will need to make as they push through the cultural shift of becoming AI-native.
1. Tailor models to your company’s unique context
You cannot scale real adoption if your AI feels generic.
Public models know the world. Your models need to know your company.
- Fine-tune internal models on your mission, customer context, and internal knowledge
- Create prompt libraries customized for different teams and use cases
- Tune temperature and tone settings based on the type of work — exploratory for brainstorming, precise for compliance and documentation
In AI-native companies, the AI speaks your language.
2. Bring AI to where work happens — make it easily accessible
Employees should not have to “go somewhere else” to use AI.
It should show up right inside the tools they are already working with.
- Embed AI into Slack, Confluence, Docs, Jira, tickets, and dashboards
- Push smart nudges, summaries, and draft suggestions into the work surface
- Make AI interaction so natural that it feels like part of getting the work done
People should not have to switch tools to feel AI’s presence. It should be woven into the work.
3. Don’t make your workforce ‘set up’ AI, offer it out of the box
Even if AI is accessible, adoption drops if it feels like extra setup work.
Employees should be able to start using AI without lifting a finger.
- Preconfigure automatic meeting note generators, document drafters, scheduling assistants
- Integrate AI helpers natively into everyday workflows
- Make getting AI support the default behavior, not a special effort
AI should not feel like another tool to install. It should feel like a natural upgrade to how work happens.
4. Treat AI-generated work as a smart way of working
Once AI is in the flow, the next hurdle is emotional.
Employees must feel proud — not guilty — when they use AI to work smarter.
- Celebrate when people use AI to automate repetitive tasks
- Normalize using AI-generated drafts, summaries, templates without shame
- Reframe craftsmanship as what you build on top of AI acceleration, not how much manual effort you spent
But changing individual behavior is only half the story.
Companies must also rethink what they actually reward.
That is where leadership needs to step in.
5. Redefine “good work”: Reward shaping and solving, not just doing
After AI enters the workflow, what counts as good performance must evolve too.
Otherwise, people will stay busy instead of moving the work forward.
- Reward employees who shape, solve, and deliver impact — not those who stay the busiest
- Encourage smart leverage and smart outcomes over manual hustle
- Train managers to value thinking, judgment, and outcome shaping — not visible grind
In the AI era, performance is not about doing more. It is about shaping better.
6. Publish data on how AI is improving speed, quality, and output
A lot of companies are talking about AI. Very few are showing how it is actually helping.
Real leadership means sharing real numbers.
- Track and report AI usage rates and adoption by function
- Measure improvements in project timelines, work quality, and operational efficiency
- Publish AI impact reporting internally — and externally when ready
The companies that show their AI progress transparently will stand out early.
7. Set up AI helpdesks and champion networks to drive hands-on adoption
Training sessions create awareness. But adoption only deepens through ongoing support and real humans helping.
- Set up internal AI helpdesks where employees can ask real questions
- Build AI champion networks inside teams to demo, coach, and share best practices
- Run workshops, demo days, and success story showcases regularly
People copy what they see working around them, not what they hear about once a year.
8. Create simple, public rules for how AI can be used inside the company
Fear and confusion slow down adoption. AI is still an emerging tech and is largely a black box for most of your employees Clear, lightweight rules around how to use AI can build trust and confidence in them to use it the right way.
- Define what kinds of data can go into public AI tools
- Clarify how and when AI-generated outputs should be verified before public/customer exposure
- Encourage responsible experimentation inside clearly communicated boundaries
Good governance is not about slowing down. It is about clearing the runway for smart risk-taking.
9. Track weekly AI usage across teams, not just employee training data
Only after the environment is ready should you start tracking serious adoption.
- Measure how many employees are using AI tools weekly to get real work done
- Break down usage by domain — engineering, marketing, design, sales
- Look for not just surface use (drafting messages) but deep integration into workflows (solving problems, building faster, making better decisions)
If you cannot see how usage is evolving, you cannot help it grow.
This shift will take a while to become normalized across the tech industry. A lot of experienced folks will need to change and update their own way of working. Eventually it will trickle down into policies and culture, and become the de-facto way companies operate and build.
AI-native companies will – |
---|
Build internal AI models trained on your company’s real knowledge |
Bring AI to where work happens and make it instantly accessible |
Set up AI assistants out of the box with no setup or friction |
Treat AI-generated work as a smart way of working |
Redefine good work to reward shaping and solving, not just doing |
Publish real numbers on how AI is improving speed, quality, and output |
Set up live AI helpdesks and champion networks to drive hands-on adoption |
Create simple, public rules for how AI can be used inside the company |
Track weekly AI usage across teams, not just training numbers |
It will be interesting to witness such a change first-hand though. The interesting part about this is that such transformations give us an opportunity to try and challenge the older ways of doing things. It’s time to run experiments on how we do the work itself. There are no established methods anymore, and that’s a lot of fun.